Using AI based diffusive size factors for extracted networks¶
This notebook illustrates the use of the deep learning based diffusive conductance algorithm decribed here. PoreSpy’s diffusive_size_factor_AI
includes the steps for predicting the diffusive size factors of the conduit images. Note that the diffusive conductance of the conduits can be then calculated by multiplying the size factor by diffusivity of the phase. The function takes in the images of segmented porous medium and returns an array of diffusive size factors for all conduits in the image. Therefore, the framework can be applied to both one conduit image as well as a segmented image of porous medium:
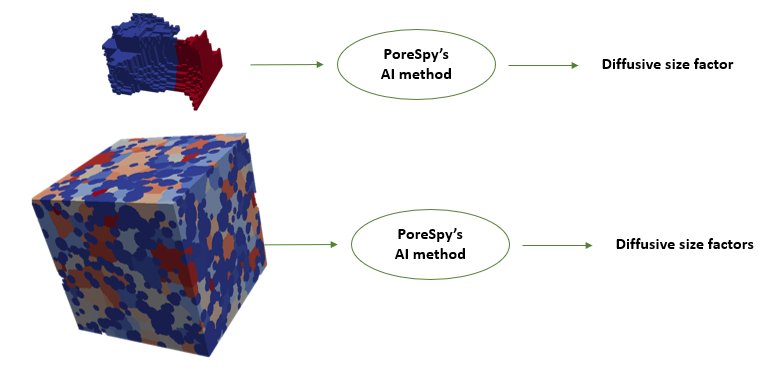
Trained model and supplementary materials¶
To use the diffusive_size_factor_AI
, the trained model, and training data distribution are required. The AI model files and additional files used in this example are available here. The folder contains following files:
Trained model weights: This file includes only weights of the deep learning layers. To use this file, the Resnet50 model structure must be built first.
Trained data distribution: This file will be used in denormalizing predicted values based on normalized transform applied on training data. The denormalizing step is included in
diffusive_size_factor_AI
method.Finite difference diffusive conductance: This file is used in this example to compare the prediction results with finite difference method for segmented regions. Note: Finite difference-based size factors can be calculated using PoreSpy’s
diffusive_size_factor_DNS
method.
Let’s download the tensorflow
files required to run this notebook:
try:
import tensorflow as tf
except ImportError:
!pip install tensorflow
try:
import sklearn
except ImportError:
!pip install scikit-learn
import os
if not os.path.exists("sf-model-lib"):
!git clone https://github.com/PMEAL/sf-model-lib
2024-06-10 03:09:56.172564: I external/local_tsl/tsl/cuda/cudart_stub.cc:32] Could not find cuda drivers on your machine, GPU will not be used.
2024-06-10 03:09:56.175750: I external/local_tsl/tsl/cuda/cudart_stub.cc:32] Could not find cuda drivers on your machine, GPU will not be used.
2024-06-10 03:09:56.213321: I tensorflow/core/platform/cpu_feature_guard.cc:210] This TensorFlow binary is optimized to use available CPU instructions in performance-critical operations.
To enable the following instructions: AVX2 FMA, in other operations, rebuild TensorFlow with the appropriate compiler flags.
2024-06-10 03:09:56.969404: W tensorflow/compiler/tf2tensorrt/utils/py_utils.cc:38] TF-TRT Warning: Could not find TensorRT
Also, since the model weights have been stored in chunks, they need to be recombined first:
import importlib
h5tools = importlib.import_module("sf-model-lib.h5tools")
DIR_WEIGHTS = "sf-model-lib/diffusion"
fname_in = [f"{DIR_WEIGHTS}/model_weights_part{j}.h5" for j in [0, 1]]
h5tools.combine(fname_in, fname_out=f"{DIR_WEIGHTS}/model_weights.h5")
Note that to use diffusive_size_factor_AI
, Scikit-learn and Tensorflow must be installed. Import necessary packages and the AI model:
import os
import warnings
import h5py
import numpy as np
import openpnm as op
import porespy as ps
import scipy as sp
from matplotlib import pyplot as plt
from sklearn.metrics import r2_score
ps.visualization.set_mpl_style()
warnings.filterwarnings("ignore")
path = "./sf-model-lib/diffusion"
path_train = os.path.join(path, 'g_train_original.hdf5')
path_weights = os.path.join(path, 'model_weights.h5')
g_train = h5py.File(path_train, 'r')['g_train'][()]
model = ps.networks.create_model()
model.load_weights(path_weights)
[03:09:58] ERROR PARDISO solver not installed, run `pip install pypardiso`. Otherwise, _workspace.py:56 simulations will be slow. Apple M chips not supported.
---------------------------------------------------------------------------
ValueError Traceback (most recent call last)
Cell In[3], line 18
16 path_weights = os.path.join(path, 'model_weights.h5')
17 g_train = h5py.File(path_train, 'r')['g_train'][()]
---> 18 model = ps.networks.create_model()
19 model.load_weights(path_weights)
File ~/work/porespy/porespy/src/porespy/networks/_size_factors.py:614, in create_model()
612 from tensorflow.keras.optimizers import Adam
613 model = _resnet3d()
--> 614 model.compile(loss='mse', optimizer=Adam(lr=1e-4), metrics=['mse'])
615 return model
File /opt/hostedtoolcache/Python/3.10.14/x64/lib/python3.10/site-packages/keras/src/optimizers/adam.py:62, in Adam.__init__(self, learning_rate, beta_1, beta_2, epsilon, amsgrad, weight_decay, clipnorm, clipvalue, global_clipnorm, use_ema, ema_momentum, ema_overwrite_frequency, loss_scale_factor, gradient_accumulation_steps, name, **kwargs)
43 def __init__(
44 self,
45 learning_rate=0.001,
(...)
60 **kwargs,
61 ):
---> 62 super().__init__(
63 learning_rate=learning_rate,
64 name=name,
65 weight_decay=weight_decay,
66 clipnorm=clipnorm,
67 clipvalue=clipvalue,
68 global_clipnorm=global_clipnorm,
69 use_ema=use_ema,
70 ema_momentum=ema_momentum,
71 ema_overwrite_frequency=ema_overwrite_frequency,
72 loss_scale_factor=loss_scale_factor,
73 gradient_accumulation_steps=gradient_accumulation_steps,
74 **kwargs,
75 )
76 self.beta_1 = beta_1
77 self.beta_2 = beta_2
File /opt/hostedtoolcache/Python/3.10.14/x64/lib/python3.10/site-packages/keras/src/backend/tensorflow/optimizer.py:22, in TFOptimizer.__init__(self, *args, **kwargs)
21 def __init__(self, *args, **kwargs):
---> 22 super().__init__(*args, **kwargs)
23 self._distribution_strategy = tf.distribute.get_strategy()
File /opt/hostedtoolcache/Python/3.10.14/x64/lib/python3.10/site-packages/keras/src/optimizers/base_optimizer.py:37, in BaseOptimizer.__init__(self, learning_rate, weight_decay, clipnorm, clipvalue, global_clipnorm, use_ema, ema_momentum, ema_overwrite_frequency, loss_scale_factor, gradient_accumulation_steps, name, **kwargs)
33 warnings.warn(
34 "Argument `decay` is no longer supported and will be ignored."
35 )
36 if kwargs:
---> 37 raise ValueError(f"Argument(s) not recognized: {kwargs}")
39 if name is None:
40 name = auto_name(self.__class__.__name__)
ValueError: Argument(s) not recognized: {'lr': 0.0001}
Create test image¶
We can create a 3D image using PoreSpy’s poly_disperese_spheres
generator:
Extract the network¶
We then extract the pore network of the porous medium image using PoreSpy’s snow2
algorithm. snow2
returns the segmented image of the porous medium as well as extracted network data.
snow = ps.networks.snow2(im, boundary_width=0, parallelization=None)
regions = snow.regions
net = snow.network
Apply diffusive_size_factor_AI¶
AI_based diffusive size factors of conduits in the extracted network can then be calculated applying diffusive_size_factor_AI
on the segmented regions. We can then define throat.diffusive_size_factor_AI
property and assign the predicted size_factor to this property.
conns = net['throat.conns']
size_factors = ps.networks.diffusive_size_factor_AI(regions,
model=model,
g_train=g_train,
throat_conns=conns)
net['throat.diffusive_size_factor_AI'] = size_factors
47/47 [==============================] - 42s 841ms/step
The resulting network can then be imported to OpenPNM for later use such as diffusive mass transport simulations problems. Let’s visualize the network:
Compare with finite difference method¶
Now that the extracted network includes AI_based diffusive size factor data, we can use the network to compare the accuracy of diffusive_size_factor_AI
, shape factor method,and geometry method (no shape factor) in contrast to finite difference method. Assuming a generic phase with diffusivity of 1, the diffusive conductance of the conduits will be equal to their diffusive size factors. The diffusive conductance of the conduits can be calculated using OpenPNM’s generic_diffusive method. The diffusive conductance of the conduits using shape factor based method assuming cones and cylinders shapes for pores and throats can be calculated as follows:
print(pn)
══════════════════════════════════════════════════════════════════════════════
net : <openpnm.network.Network at 0x7f0256f989a0>
――――――――――――――――――――――――――――――――――――――――――――――――――――――――――――――――――――――――――――――
# Properties Valid Values
――――――――――――――――――――――――――――――――――――――――――――――――――――――――――――――――――――――――――――――
2 throat.conns 744 / 744
3 pore.coords 199 / 199
4 pore.region_label 199 / 199
5 pore.phase 199 / 199
6 throat.phases 744 / 744
7 pore.region_volume 199 / 199
8 pore.equivalent_diameter 199 / 199
9 pore.local_peak 199 / 199
10 pore.global_peak 199 / 199
11 pore.geometric_centroid 199 / 199
12 throat.global_peak 744 / 744
13 pore.inscribed_diameter 199 / 199
14 pore.extended_diameter 199 / 199
15 throat.inscribed_diameter 744 / 744
16 throat.total_length 744 / 744
17 throat.direct_length 744 / 744
18 throat.perimeter 744 / 744
19 pore.volume 199 / 199
20 pore.surface_area 199 / 199
21 throat.cross_sectional_area 744 / 744
22 throat.equivalent_diameter 744 / 744
23 throat.diffusive_size_factor_AI 744 / 744
――――――――――――――――――――――――――――――――――――――――――――――――――――――――――――――――――――――――――――――
# Labels Assigned Locations
――――――――――――――――――――――――――――――――――――――――――――――――――――――――――――――――――――――――――――――
2 pore.all 199
3 throat.all 744
――――――――――――――――――――――――――――――――――――――――――――――――――――――――――――――――――――――――――――――
pn['pore.diameter'] = pn['pore.inscribed_diameter']
pn['throat.diameter'] = pn['throat.inscribed_diameter']
pn['throat.coords'] = pn['throat.global_peak']
pn.add_model(propname='throat.length',
model=op.models.geometry.throat_length.hybrid_cones_and_cylinders)
pn.add_model(propname='throat.diffusive_size_factors',
model=op.models.geometry.diffusive_size_factors.cones_and_cylinders,)
phase = op.phase.Phase(network=pn)
phase['pore.diffusivity'] = 1
phase['throat.diffusivity'] = 1
phase.add_model(propname='throat.diffusive_conductance',
model=op.models.physics.diffusive_conductance.generic_diffusive)
g_SF = np.copy(phase['throat.diffusive_conductance'])
To find the diffusive conductance of the conduit using geometry method (no shape factor) we assume cylindrical pores and throats:
cn = pn.conns
L1, Lt, L2 = pn['pore.diameter'][
cn[:, 0]] / 2, pn['throat.length'], pn['pore.diameter'][cn[:, 1]] / 2
D1, Dt, D2 = pn['pore.diameter'][
cn[:, 0]], pn['throat.diameter'], pn['pore.diameter'][cn[:, 1]]
A1, At, A2 = np.pi * D1**2 / 4, np.pi * Dt**2 / 4, np.pi * D2**2 / 4
g_Geo = 1 / (L1 / A1 + L2 / A2 + Lt / At)
The diffusive conductance of the conduit using AI-based method:
phase.add_model(propname='throat.diffusive_conductance',
model=op.models.physics.diffusive_conductance.generic_diffusive,
size_factors='throat.diffusive_size_factor_AI')
g_AI = np.copy(phase['throat.diffusive_conductance'])
The finite difference-based diffusive size factors were calculated using PoreSpy’s size factor method diffusive_size_factor_DNS
. However, due to the long runtime of the DNS function the results were saved in the example data folder and used in this example. The Following code was used to estimate the finite difference-based values using PoreSpy:
g_FD = ps.networks.diffusive_size_factor_DNS(regions, conns)
Now let’s compare the diffusive conductance calculated from geometry-based method, shape factor based-method, and AI-based method with reference finite difference method:
fname = os.path.join(path, 'g_finite_difference120-phi7.hdf5')
g_FD = h5py.File(fname, 'r')['g_finite_difference'][()]
max_val = np.max([g_FD, g_AI, g_Geo, g_SF])
fig, ax = plt.subplots(1, 3, figsize=[10, 4])
ax[0].plot(g_FD, g_Geo, '*', [0, max_val], [0, max_val], 'r')
ax[0].set_xlim([0, max_val])
ax[0].set_ylim([0, max_val])
ax[0].set_xlabel('finite difference $g_d$')
ax[0].set_ylabel('geometry based $g_d$')
ax[0].set_title('$R^2$ = ' + str(np.round(r2_score(g_FD, g_Geo), 2)))
ax[1].plot(g_FD, g_SF, '*', [0, max_val], [0, max_val], 'r')
ax[1].set_xlim([0, max_val])
ax[1].set_ylim([0, max_val])
ax[1].set_xlabel('finite difference $g_d$')
ax[1].set_ylabel('shape factor based $g_d$')
ax[1].set_title('$R^2$ = ' + str(np.round(r2_score(g_FD, g_SF), 2)))
ax[2].plot(g_FD, g_AI, '*', [0, max_val], [0, max_val], 'r')
ax[2].set_xlim([0, max_val])
ax[2].set_ylim([0, max_val])
ax[2].set_xlabel('finite difference $g_d$')
ax[2].set_ylabel('AI based $g_d$')
ax[2].set_title(r'$R^2$ = ' + str(np.round(r2_score(g_FD, g_AI), 2)));
As shown in the scatter plots, the AI-based diffusive conductance method predicts the conductance values with a higher accuracy than geometry-based and shape factor-based methods. A comprehensive comparison between these methods for a large dataset can be found here.