norm_to_uniform
#
Import packages#
import numpy as np
import porespy as ps
import scipy.ndimage as spim
import matplotlib.pyplot as plt
import skimage
ps.visualization.set_mpl_style()
[17:45:18] ERROR PARDISO solver not installed, run `pip install pypardiso`. Otherwise, _workspace.py:56 simulations will be slow. Apple M chips not supported.
Generate image for testing#
im = np.random.rand(200, 200)
strel = ps.tools.ps_disk(20, smooth=False)
im = spim.convolve(im, weights=strel)
fig, ax = plt.subplots(1, 2, figsize=[8, 4])
ax[0].axis(False)
ax[0].imshow(im)
ax[1].hist(im.flatten(), edgecolor='k', bins=25)
ax[1].set_xlabel('Value')
ax[1].set_ylabel('Counts');
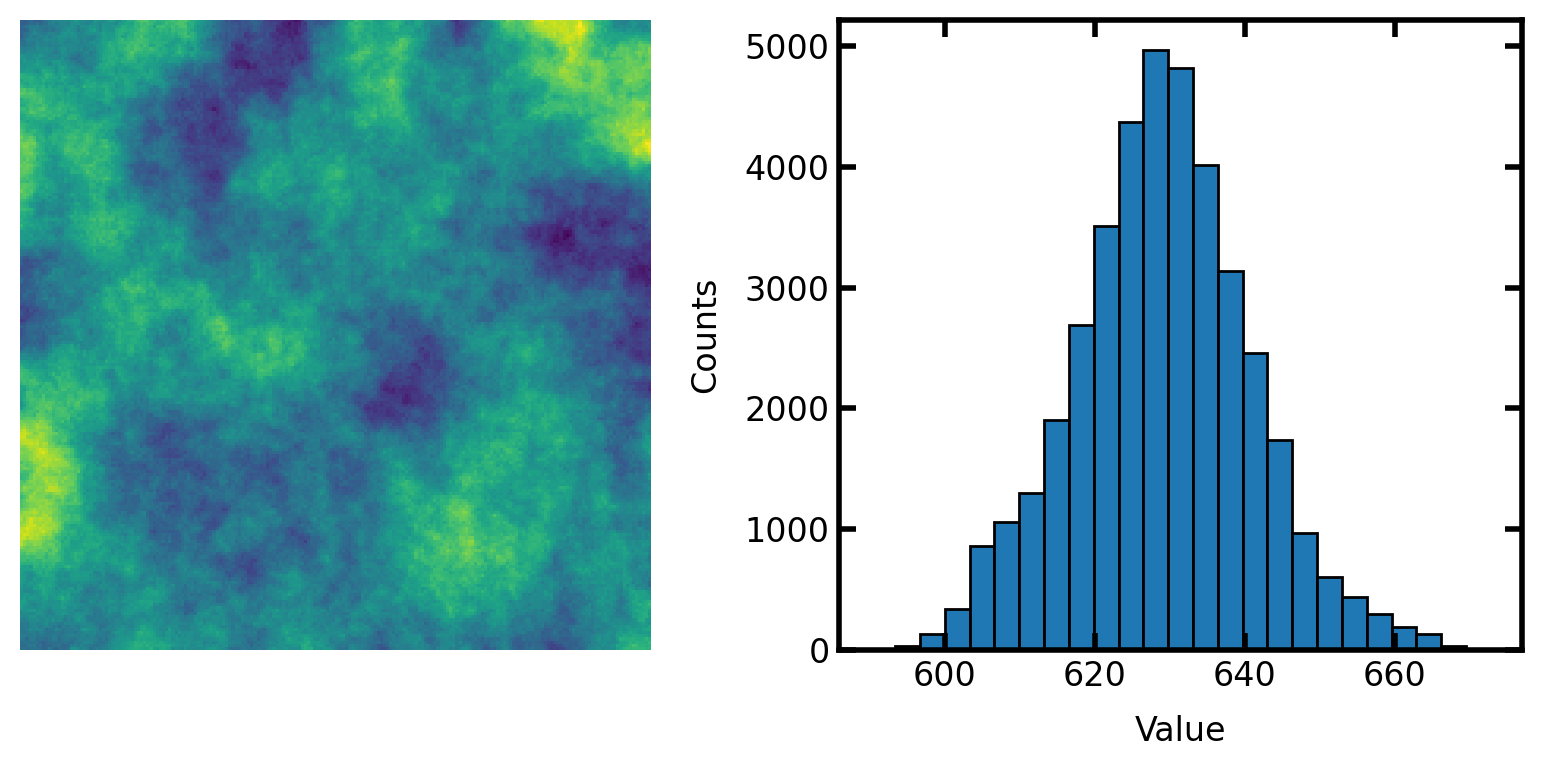
Demonstrate function#
The correlated noise field generated above has approximatetly normally distributed values. It’s not perfectly normal, but it’s pretty close. This can be converted to uniformly distributed values as follows:
im1 = ps.tools.norm_to_uniform(im=im)
fig, ax = plt.subplots(1, 2, figsize=[8, 4])
ax[0].axis(False)
ax[0].imshow(im1)
ax[1].hist(im1.flatten(), edgecolor='k', bins=25)
ax[1].set_xlabel('Value')
ax[1].set_ylabel('Counts');
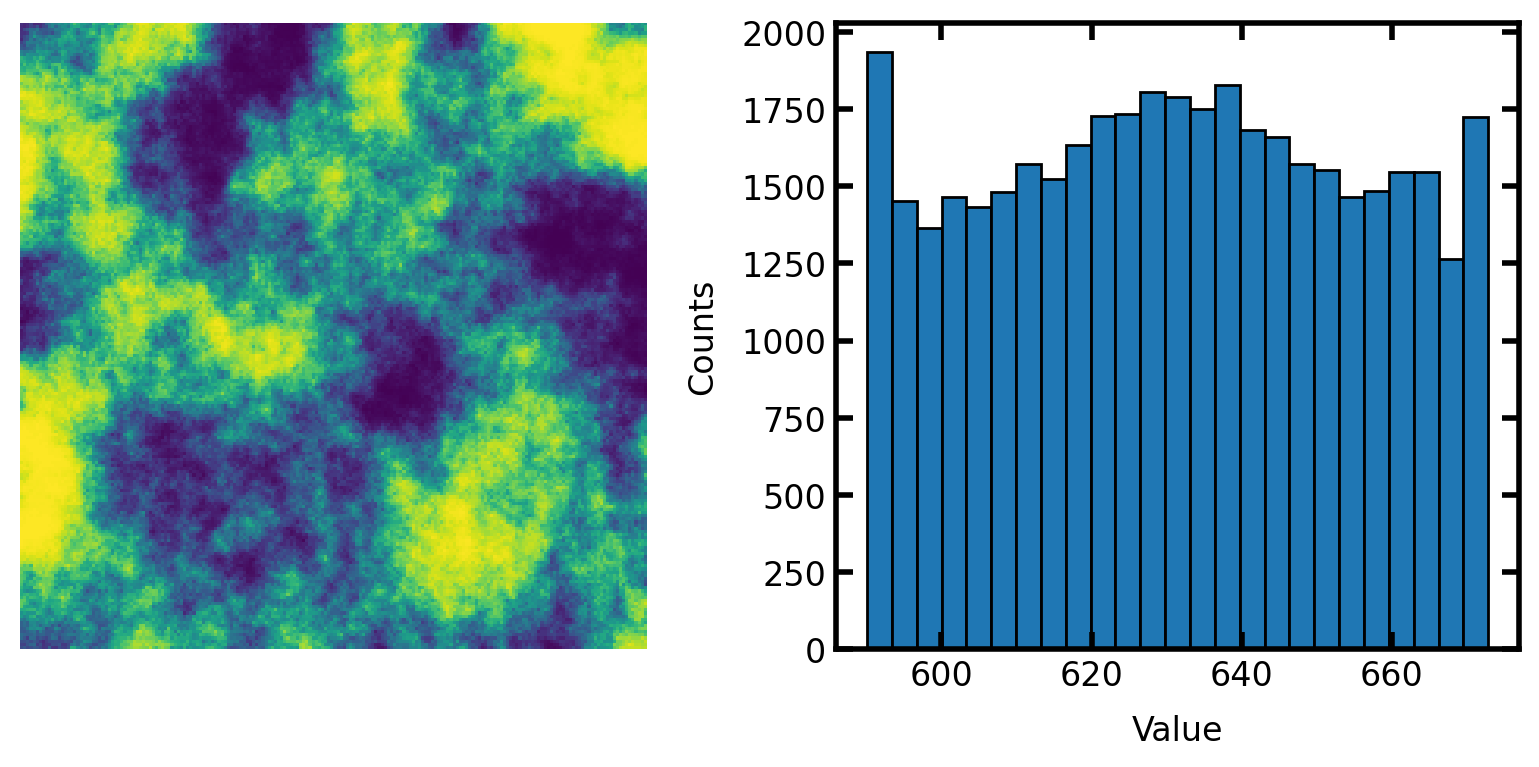
scale#
The output can be scale to a specific range:
im2 = ps.tools.norm_to_uniform(im=im, scale=[0, 1])
fig, ax = plt.subplots(1, 2, figsize=[8, 4])
ax[0].axis(False)
ax[0].imshow(im2)
ax[1].hist(im2.flatten(), edgecolor='k', bins=25)
ax[1].set_xlabel('Value')
ax[1].set_ylabel('Counts');
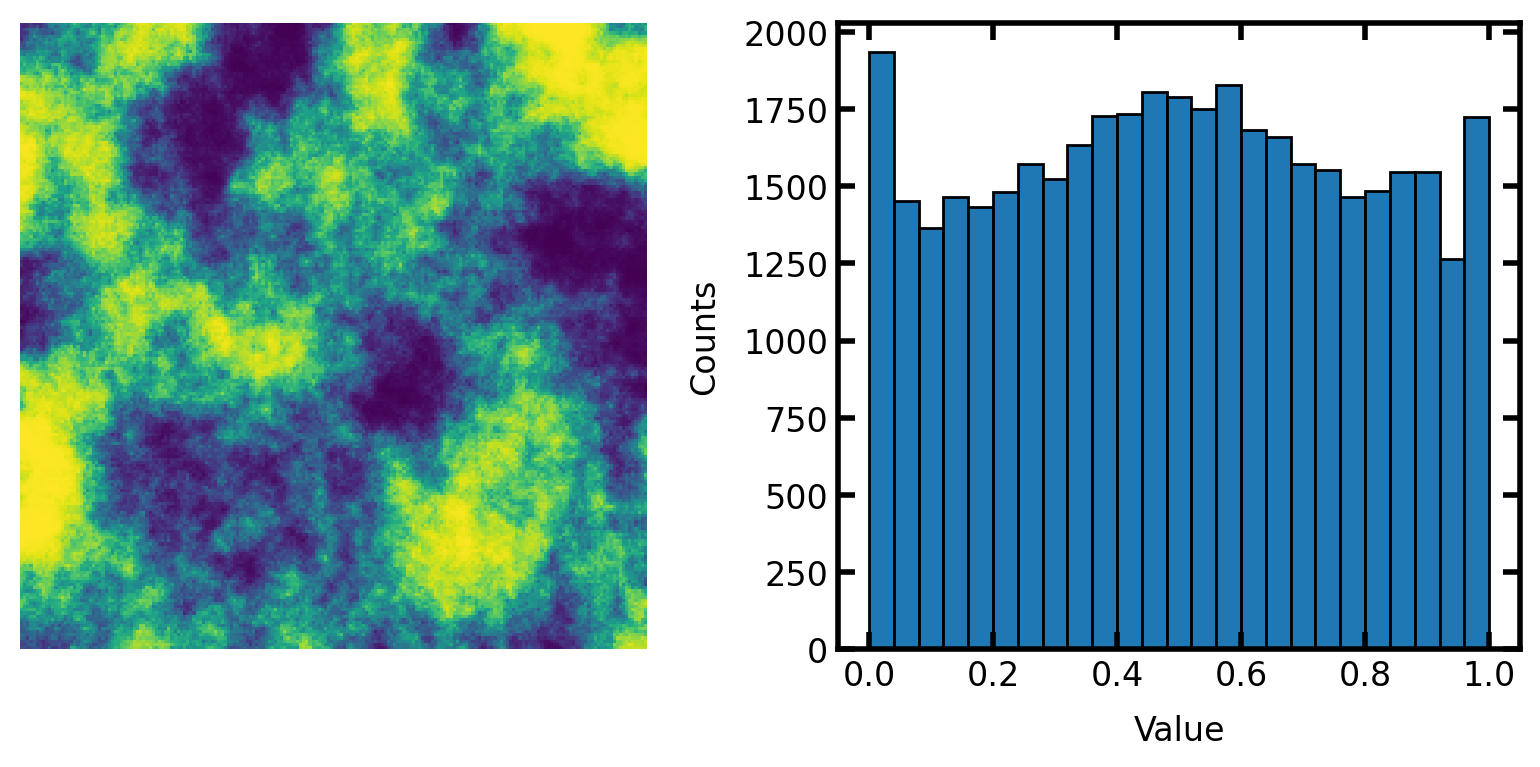